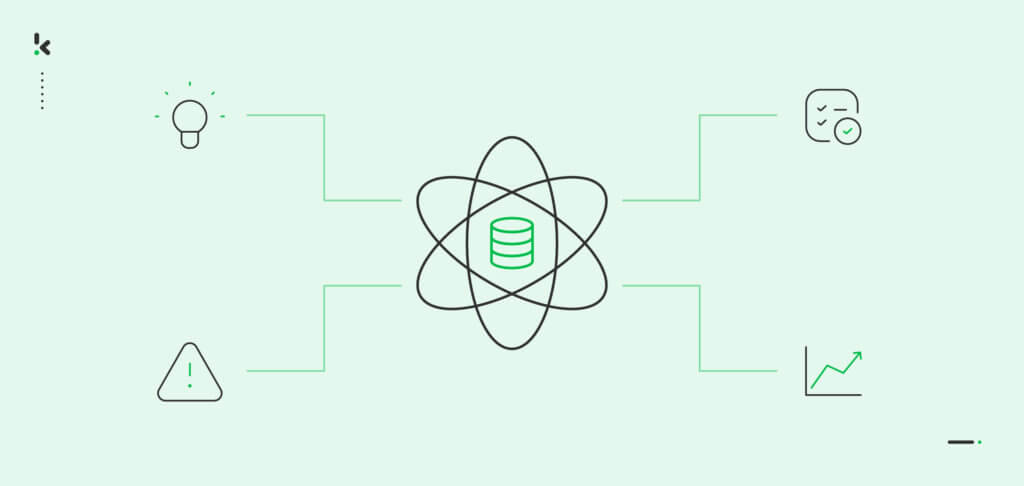
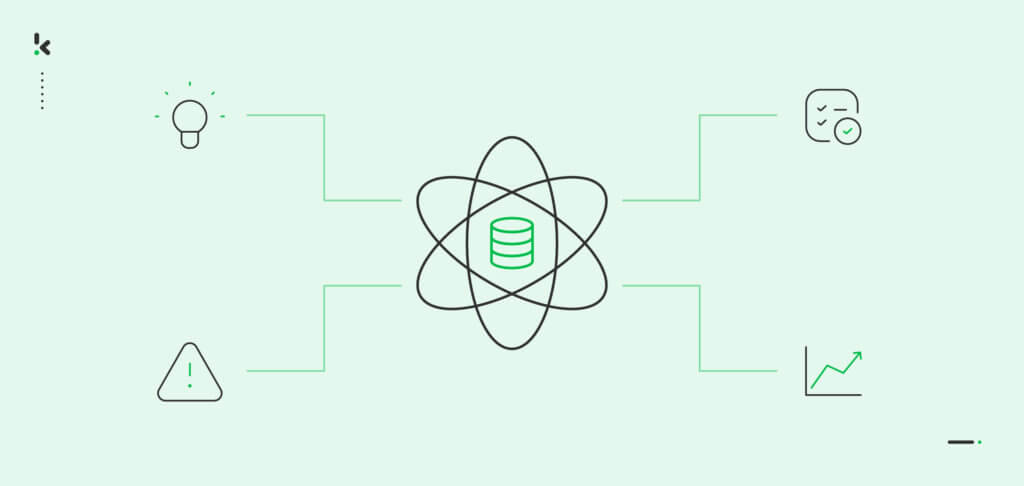
In today’s data-driven landscape, where businesses are constantly striving for innovation and growth, harnessing the power of data science has become crucial. However, embarking on a data science initiative without a solid foundation can lead to inefficiencies and wasted resources. This is where Proof of Concept (PoC) steps in as a game-changer.
A data science PoC serves as the cornerstone for the successful implementation and adoption of data-driven strategies. It allows businesses and organizations to explore the vast potential of data science in a controlled and measurable manner, paving the way for informed decision-making and successful transformations in their business operations.
But why is a PoC so important? The answer lies in mitigating risks and maximizing returns. By undertaking a Proof of Concept, you gain valuable insights into the feasibility and viability of integrating data science into your business processes. It allows you to test hypotheses, validate assumptions, and assess the impact of data-driven solutions before making significant investments.
In this blog, we will delve deeper into the world of Proof of Concept in Data Science. We’ll explore what a PoC is, common mistakes, and best practices to help you unlock the full potential of your business through data-driven decision-making.
Let’s begin!
What is a Proof of Concept?
A Proof of Concept is a preliminary demonstration or experiment designed to validate the feasibility and potential of a concept, idea, or technology before committing significant resources to its implementation. It serves as a small-scale model or prototype that aims to showcase the practicality and viability of a proposed solution.
POCs for Different Types of Projects
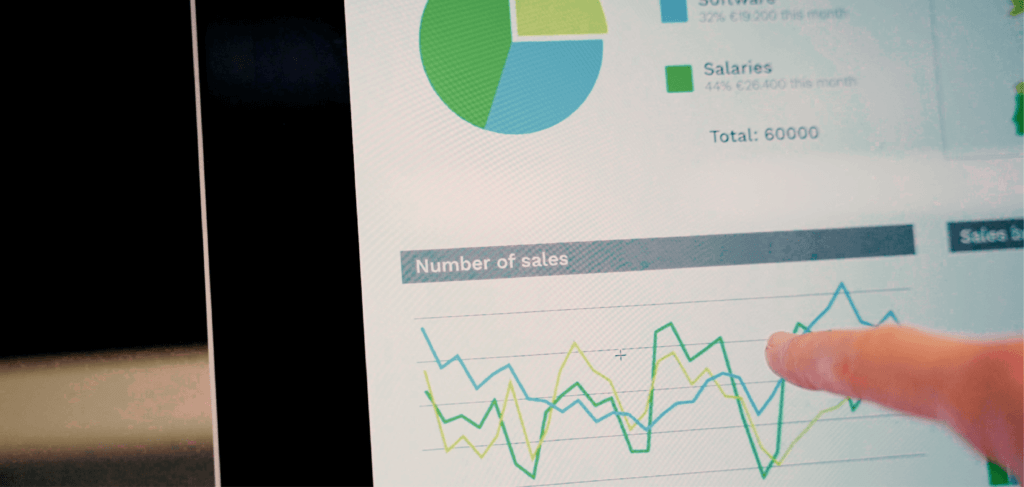
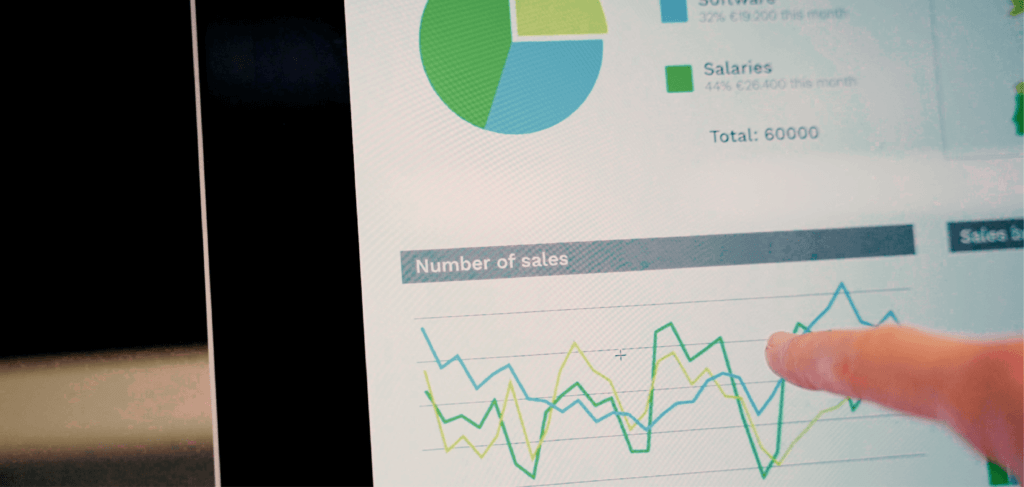
Proof of Concepts can be undertaken for a wide range of data science and analytics projects, each catering to different objectives and challenges. Some common types of projects where Proof of Concept is applied include:
- Predictive Modeling and Machine Learning: POCs can be conducted to explore the potential of predictive models and machine learning algorithms. For instance, organizations may develop a PoC to evaluate the effectiveness of a new algorithm in predicting customer churn or forecasting sales demand. By applying the algorithm to a subset of available data, the organization can gauge its accuracy and determine if it is suitable for deployment on a larger scale.
- Data Visualization and Reporting: POCs can be employed to assess different data visualization techniques and reporting frameworks. By creating prototypes and visual representations, organizations can evaluate the clarity, accessibility, and user-friendliness of their chosen tools. This helps stakeholders make informed decisions and ensures the effective communication of insights derived from complex datasets.
- Data Integration and Management: POCs are valuable when exploring data integration and management solutions. For instance, organizations may develop a PoC to test the integration of various data sources into a unified data platform or to assess the feasibility of implementing a new data governance framework. This allows organizations to identify potential challenges, such as data quality issues or compatibility problems, and make necessary adjustments before undertaking larger-scale initiatives.
However, before delving into an array of enticing Proof of Concept projects that you envision launching within your organization, it is crucial to recognize the fundamental significance of data preparation. Neglecting this critical step would jeopardize the prospects of achieving a successful PoC outcome.
The Importance of Data Preparation
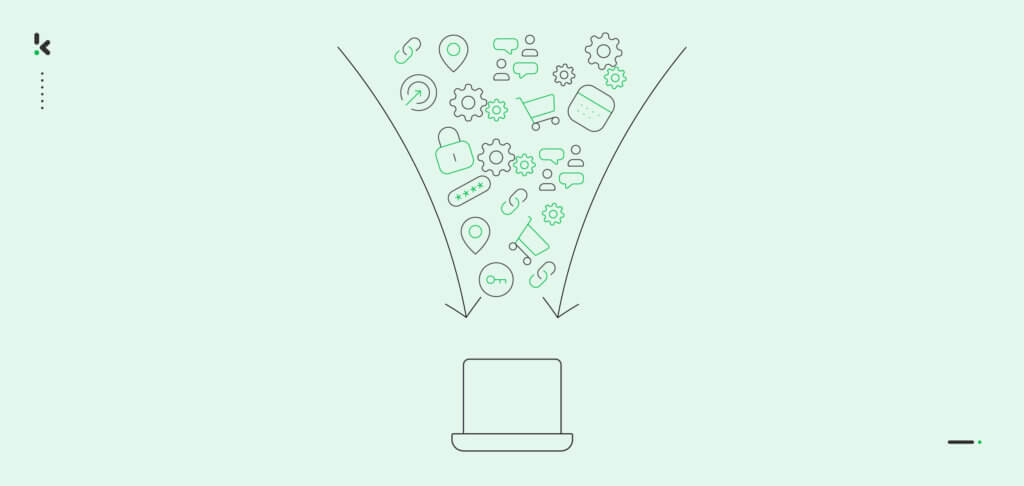
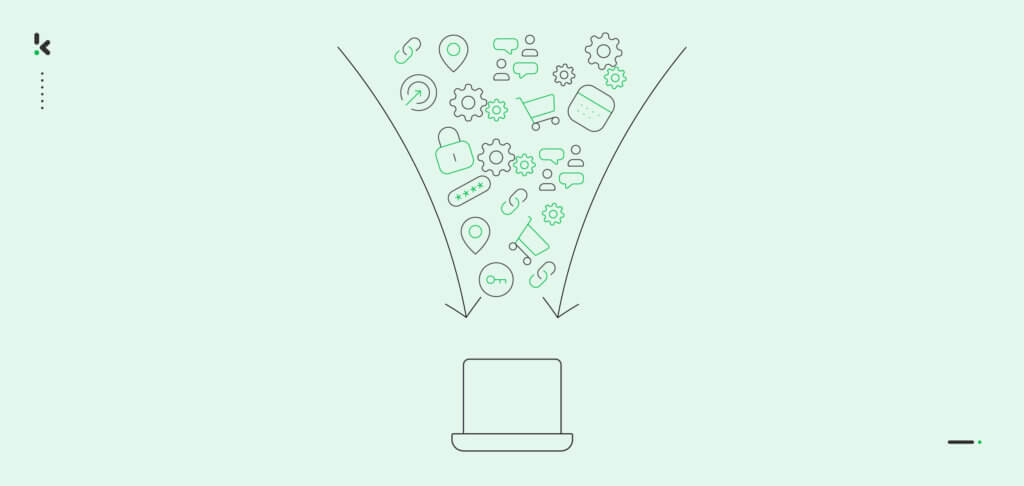
In any data science or analytics project, the success of Proof of Concepts relies heavily on the quality and suitability of the data. This is where data preparation plays a crucial role. Data preparation involves various tasks such as data cleansing, transformation, and structuring, all aimed at ensuring the data’s overall quality, consistency, and reliability. By carefully preparing the data, the integrity of the data can be enhanced and its suitability for analysis can be improved.
Here are three key reasons highlighting the importance of data preparation:
Accurate Assessments: By performing thorough data preparation, organizations can reduce the likelihood of biased results or inaccurate conclusions. Adequate data preparation allows researchers and analysts to have confidence in the validity of their findings. This ensures that the PoC accurately represents the potential outcomes of a full-scale implementation.
Identifying Data Limitations: During data preparation, analysts can identify and address limitations within the available data. This process helps uncover missing values, outliers, or inconsistent records that might affect the reliability of the analysis. By addressing these issues or considering their potential impact, organizations can improve their understanding of the project’s viability. This allows them to set realistic expectations for the complete implementation and make more informed decisions.
Sufficient Data Availability: Data preparation also involves ensuring sufficient data availability for the PoC. Insufficient data can lead to inconclusive results or inaccurate representations of the project’s potential. Organizations can leverage synthetic data to address data scarcity—an artificially generated dataset that mimics real data. By incorporating synthetic data alongside real data, organizations can enhance analyses, improve accuracy, and make more informed decisions before committing to larger investments.
Now that we have explored the various use cases for POCs in data science and acknowledged the significance of data preparation, let’s delve into the six most frequently encountered mistakes when conducting a data science PoC.
6 Most Made Mistakes when Running a Data Science PoC
Organizations often make several common mistakes during the PoC process, hindering its success. We’ll explore six prevalent mistakes in data science POCs and their impact, offering valuable insights for successful execution.
The six most commonly made mistakes when running a data science Proof of Concept are:
- Choosing an unclear use case
- Taking too much time
- Having unclear goals
- Not involving enough people
- Involving too many people
- Never reaching the production stage
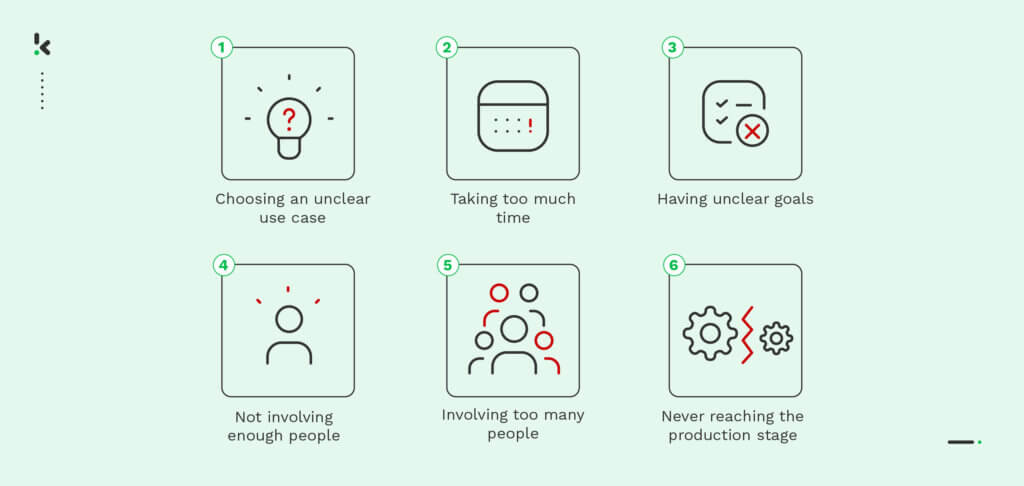
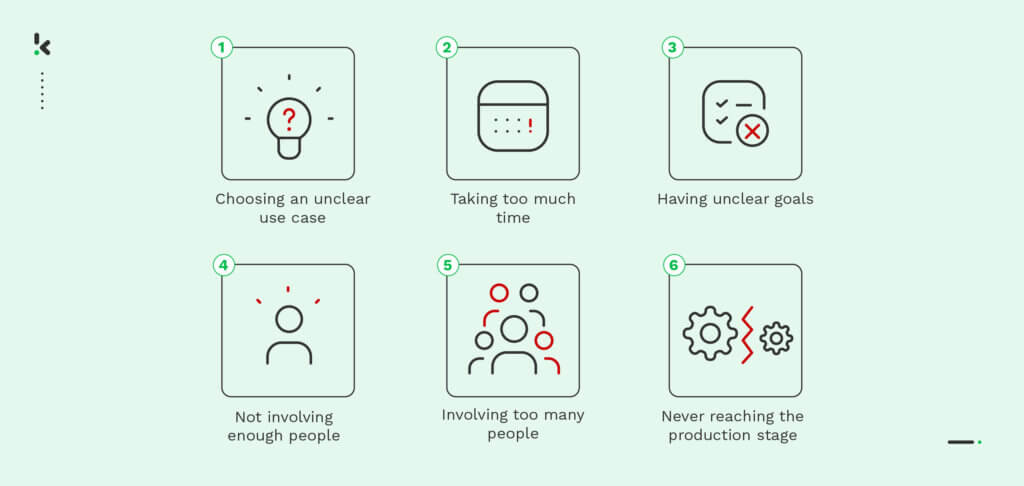
Choosing an Unclear Use Case
Selecting an unclear or ill-defined use case can lead to a lack of direction and hinder the success of a PoC. Without a clear understanding of the problem at hand or the potential value of the solution, a Proof of Concept may fail to yield meaningful insights and outcomes.
Taking too Much Time
Poor time management can be detrimental to the success of a Proof of Concept. Excessive time spent on a single PoC can delay progress and hinder the exploration of other valuable use cases, limiting the overall efficiency and impact of the initiative.
Having Unclear Goals
Unclear goals can hinder the evaluation and decision-making process during a data science PoC. Without well-defined objectives, it becomes challenging to measure the success or failure of the PoC accurately and determine the next steps.
Not Involving Enough People
Limited involvement of relevant stakeholders can lead to a narrow perspective and potentially neglect valuable insights during a PoC. Collaboration and input from a diverse group of experts are crucial to enrich the outcomes and ensure comprehensive analysis.
Involving too Many People
On the other hand, involving too many people can impede progress and decision-making during a data science Proof of Concept. Communication challenges, conflicting opinions, and difficulties in coordination may arise, hindering the efficient execution of the PoC.
Never Reaching the Production Stage
Failing to transition from the Proof of Concept stage to full production implementation is a significant mistake. When POCs remain isolated experiments, their potential impact on the organization is limited, preventing the realization of the intended benefits and outcomes.
By being aware of these common mistakes, organizations can take proactive measures to address them. This, in turn, enhances the overall effectiveness and success of their data science POCs.
Let’s now explore the essential steps you can take to ensure a successful launch of a data science PoC.
6 Steps to a Successful Data Science PoC
This chapter presents six crucial steps to enhance the success of a data science Proof of Concept. It covers the entire process, starting from selecting a practical use case to achieving production readiness. The following steps are outlined:
- Choose a practical and valuable use case
- Choose a reasonable timeframe
- Specifically define the wanted outcomes/deliverables
- Involve the right people
- Stay focused on the main deliverables
- Aim for production
Choose a Practical and Valuable Use Case
The first step in a successful data science PoC is selecting a use case that is both practical and valuable. Focus on identifying a problem or challenge that can benefit from data-driven insights and has the potential to provide significant value to the organization.
Consider factors such as the availability of relevant data, the potential impact on key business metrics, and the alignment with organizational goals and priorities. It is crucial to choose a use case that is manageable within the scope of a PoC while addressing a real and pressing business need.
3 Quick Tips
- Conduct a thorough analysis of your organization’s pain points and challenges to identify potential use cases for a PoC.
- Prioritize use cases that align with strategic objectives and have the potential to deliver tangible value.
- Ensure the availability and quality of relevant data required for the selected use case.
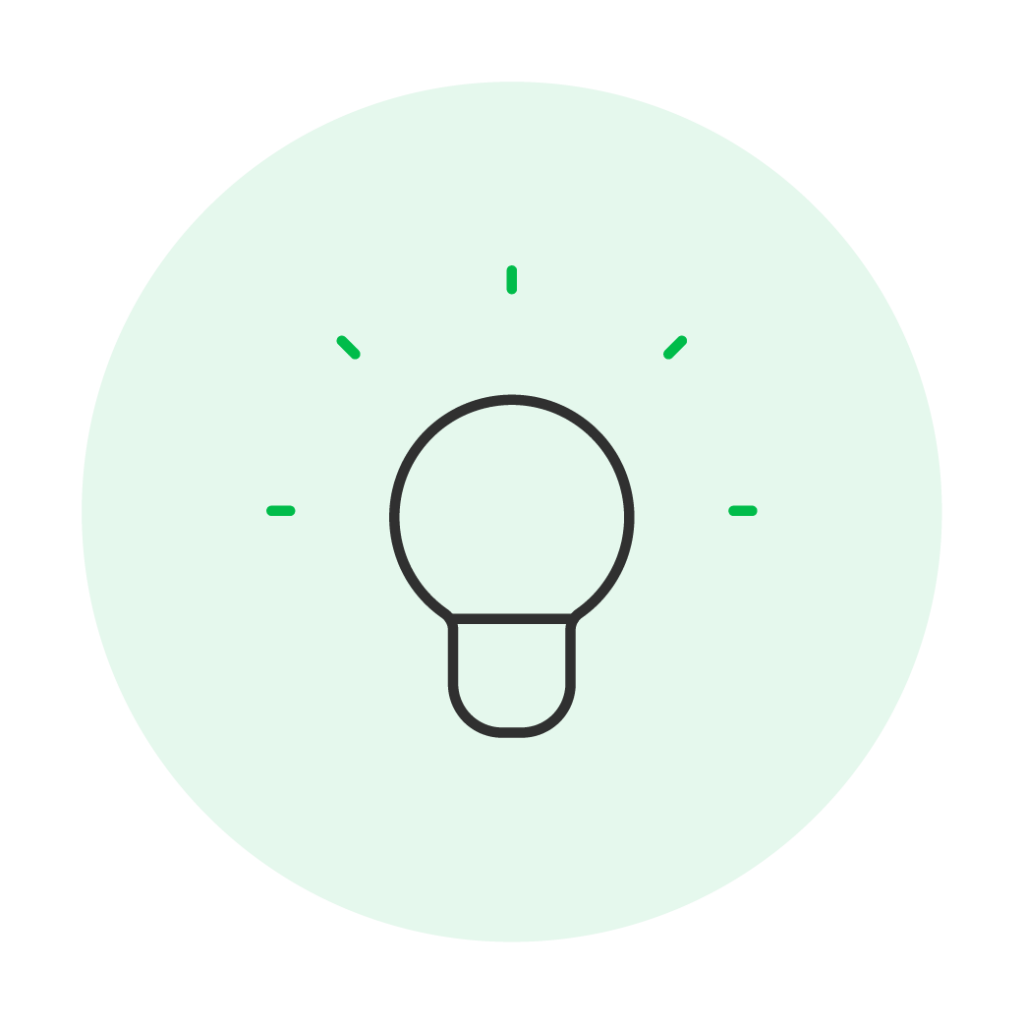
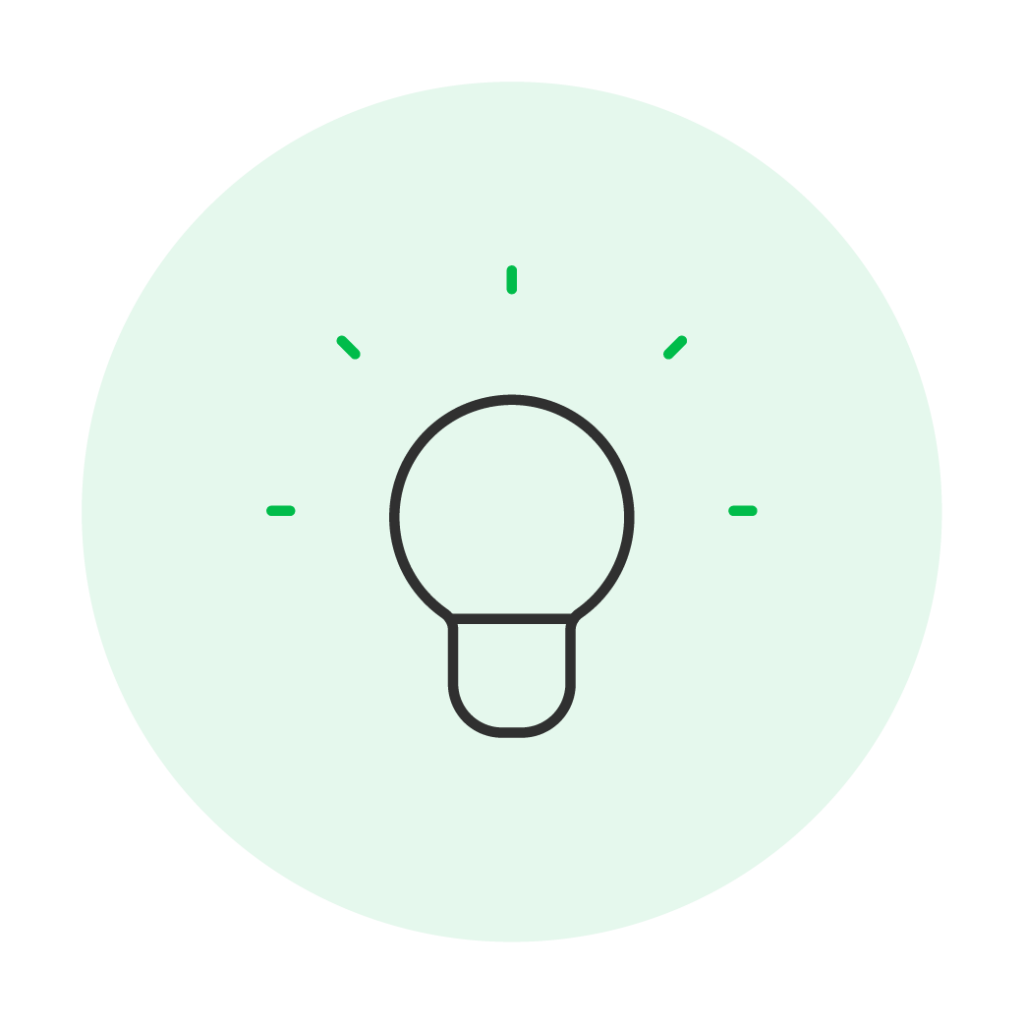
Choose a Reasonable Timeframe
Setting a reasonable timeframe is essential to ensure the efficient execution of a Proof of Concept. It is crucial to strike a balance between allowing sufficient time for exploration, experimentation, and evaluation while avoiding unnecessary delays that may hinder progress. Consider the complexity of the use case, the availability of resources, and any external constraints or deadlines when defining the timeframe for the PoC.
3 Quick Tips
- Break down the PoC into smaller milestones and allocate time for each phase, including data preparation, modeling, evaluation, and reporting.
- Set realistic deadlines for each milestone, considering the complexity of the use case and the availability of resources.
- Be flexible and open to adjusting the timeframe as the PoC progresses, based on new insights and challenges encountered.
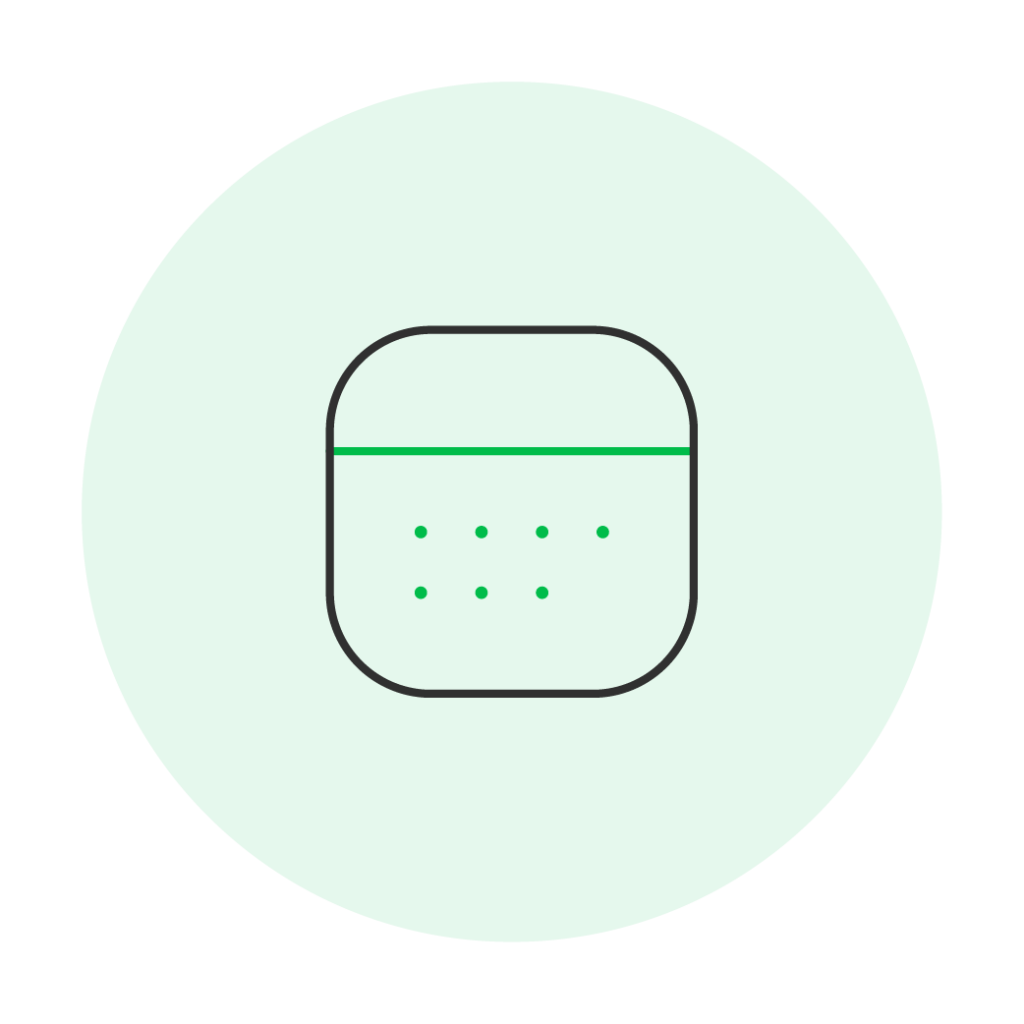
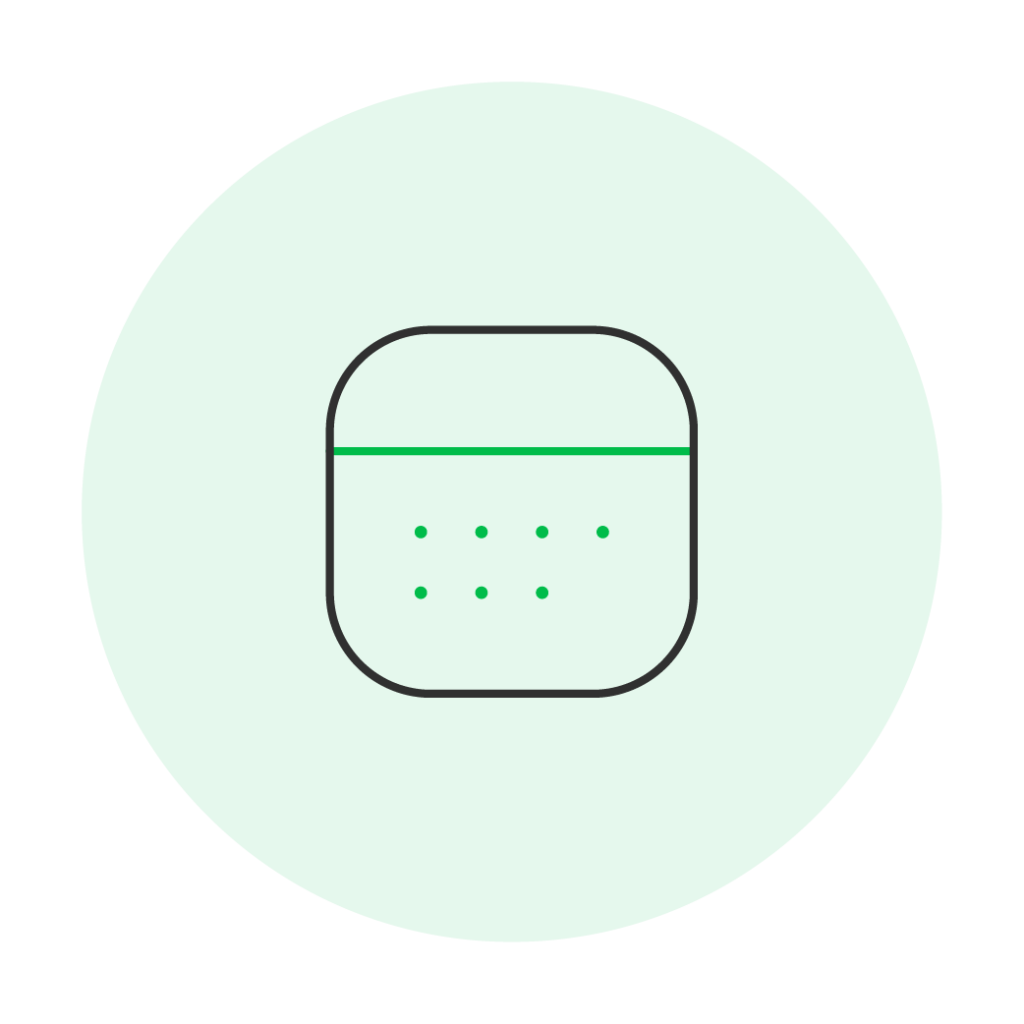
Specifically Define the Wanted Outcomes/Deliverables
Clearly defining the desired outcomes and deliverables of the data science PoC is crucial for setting expectations and measuring success. Collaborate with key stakeholders and subject matter experts to identify the specific metrics, KPIs, or objectives that the PoC aims to achieve. This clarity helps in aligning efforts, focusing resources, and evaluating the effectiveness of the proposed solution.
3 Quick Tips
- Engage with stakeholders and domain experts to understand their expectations and define measurable success criteria for the PoC.
- Clearly articulate the key metrics or objectives that the PoC should address, such as improved accuracy, cost reduction, increased efficiency, or enhanced customer satisfaction.
- Ensure that the defined outcomes align with the overall goals and priorities of the organization.
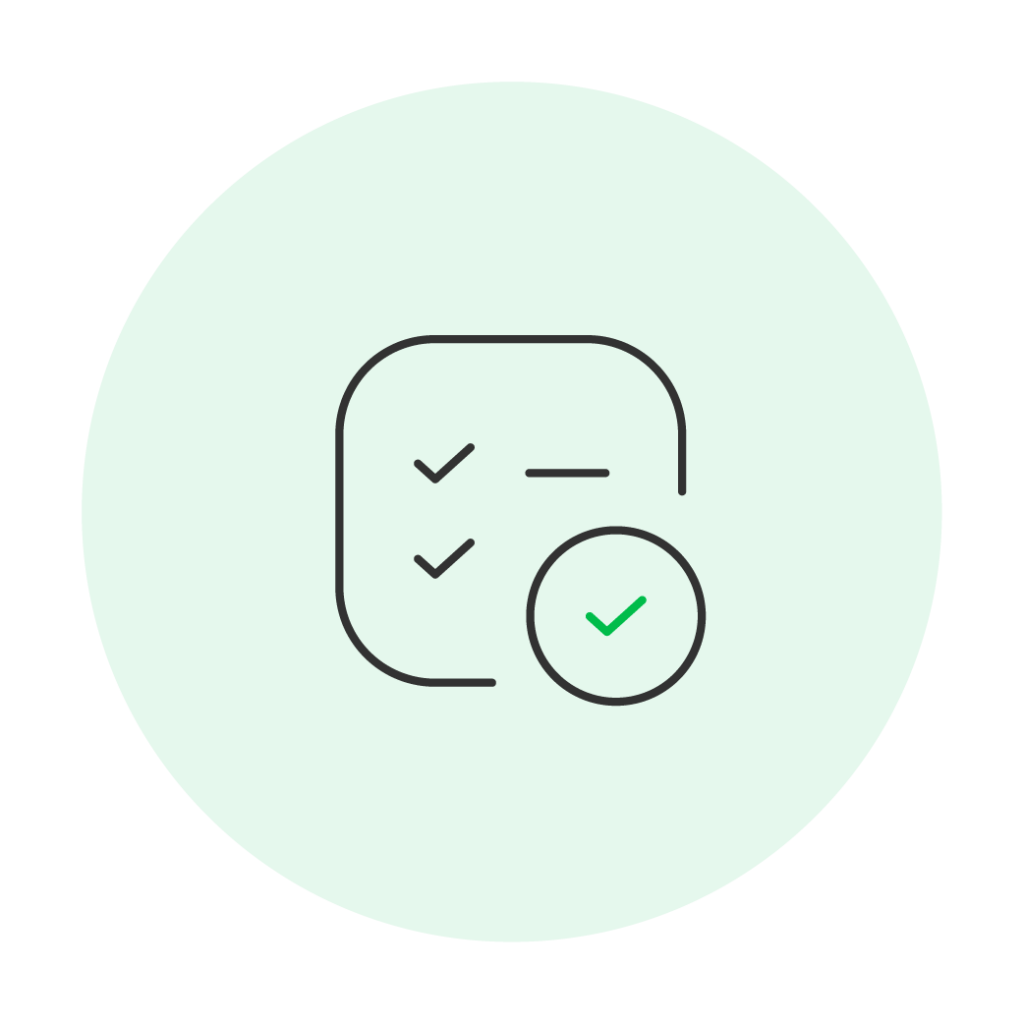
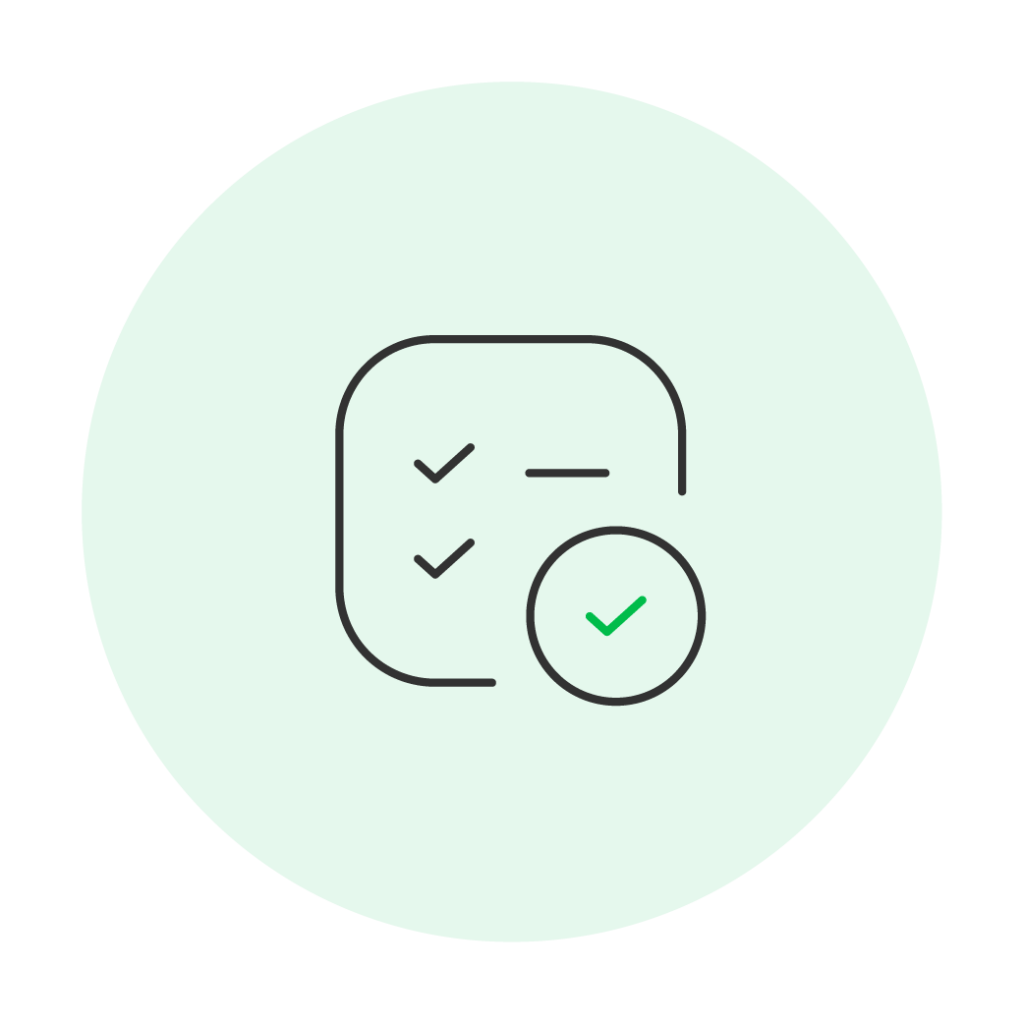
Involve the Right People
To maximize the chances of success, it is essential to involve the right people throughout the data science Proof of Concept. Collaborate with subject matter experts, data engineers, data scientists, and other relevant stakeholders who possess the necessary domain knowledge and technical expertise.
Their involvement ensures a comprehensive understanding of the problem domain, effective data preparation, accurate modeling, and insightful interpretation of the results.
3 Quick Tips
- Form a multidisciplinary team that includes domain experts, data scientists, data engineers, and business stakeholders.
- Foster a collaborative environment where team members can freely exchange ideas, share insights, and provide feedback.
- Leverage the expertise of team members to validate assumptions, guide feature selection, and interpret the results in the context of the business problem.
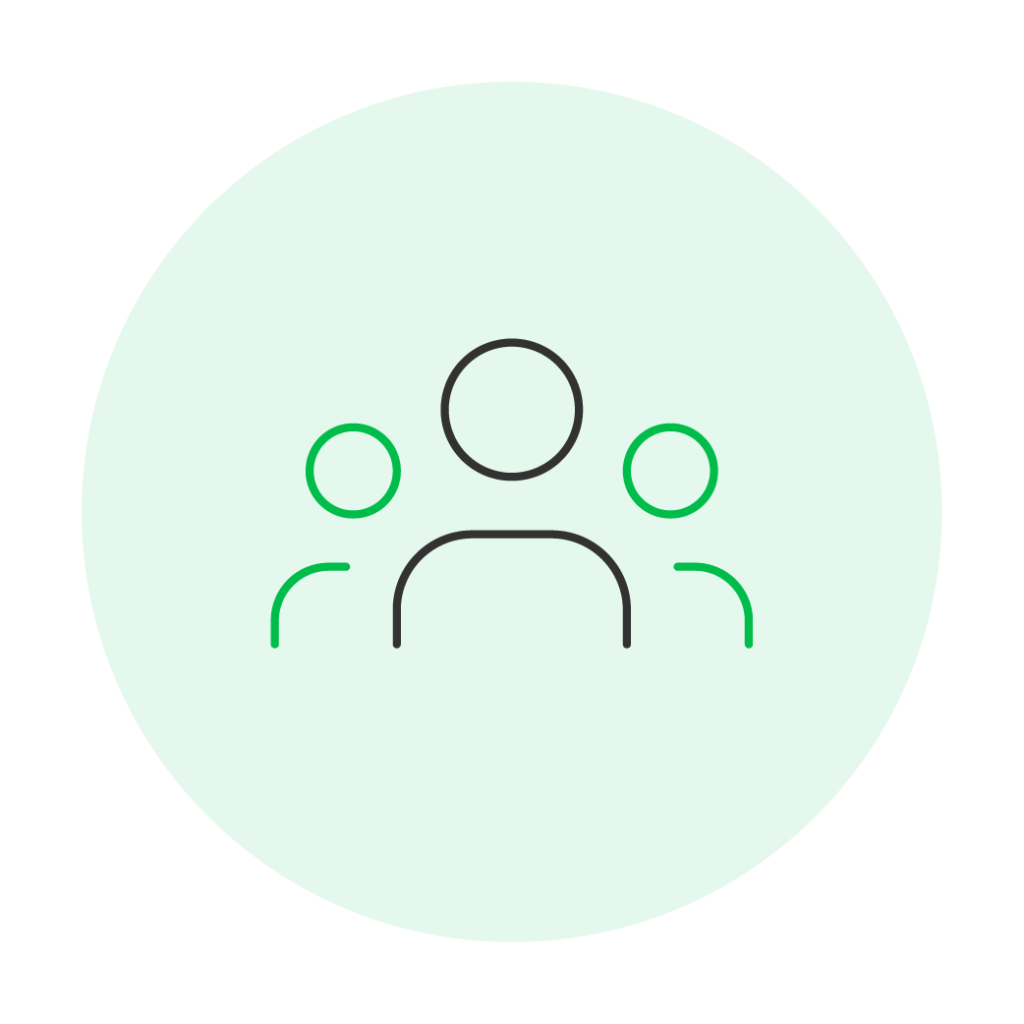
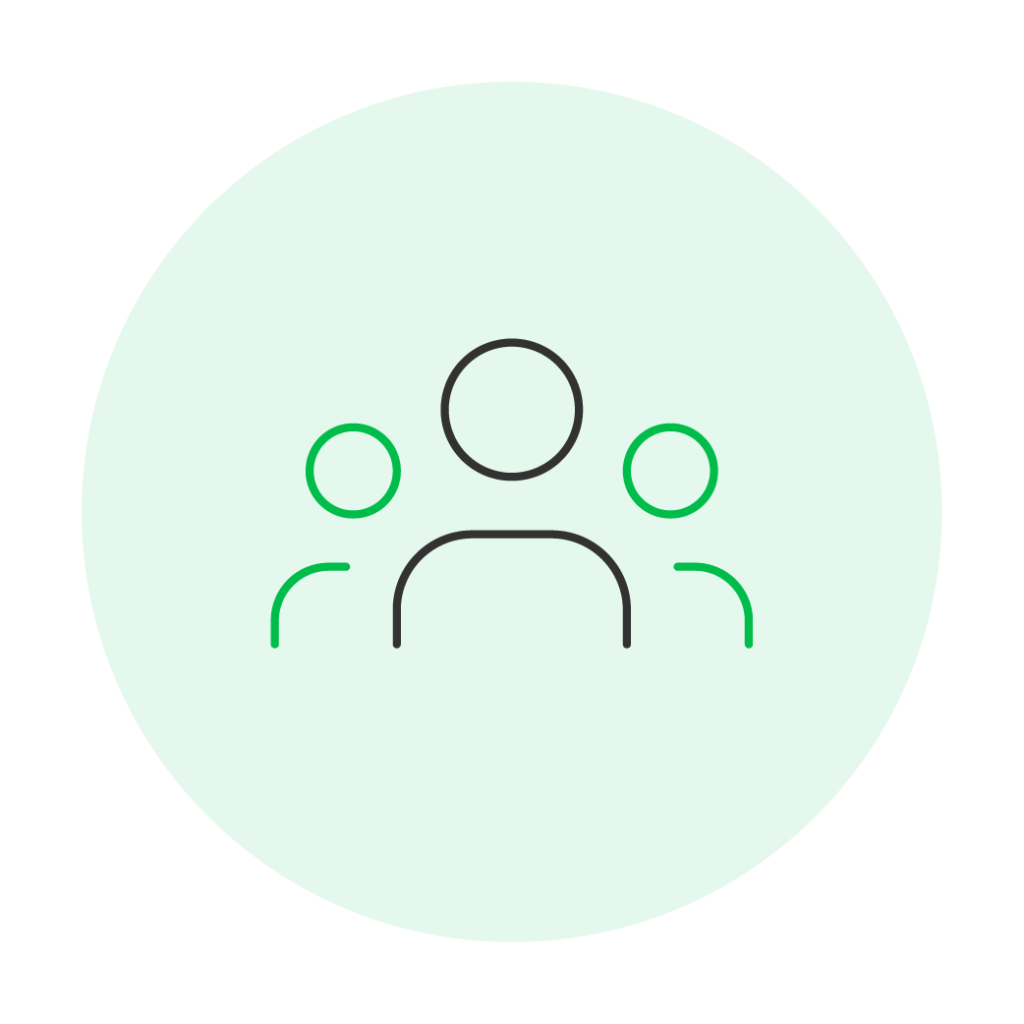
Stay Focused on the Main Deliverables
During the course of the PoC, it is crucial to stay focused on the main deliverables defined in the earlier stages. While it’s natural to encounter unexpected insights and opportunities, it’s important to avoid scope creep and maintain alignment with the initial goals. Regularly revisit the defined outcomes and keep the PoC on track to provide actionable insights and evidence of feasibility.
3 Quick Tips
- Continuously refer to the defined outcomes and deliverables to ensure the PoC stays focused.
- Regularly communicate with stakeholders to keep them informed of progress and seek their input to stay aligned with their expectations.
- Document and track any significant deviations or modifications to the initial goals, ensuring they are well-justified and supported by evidence.
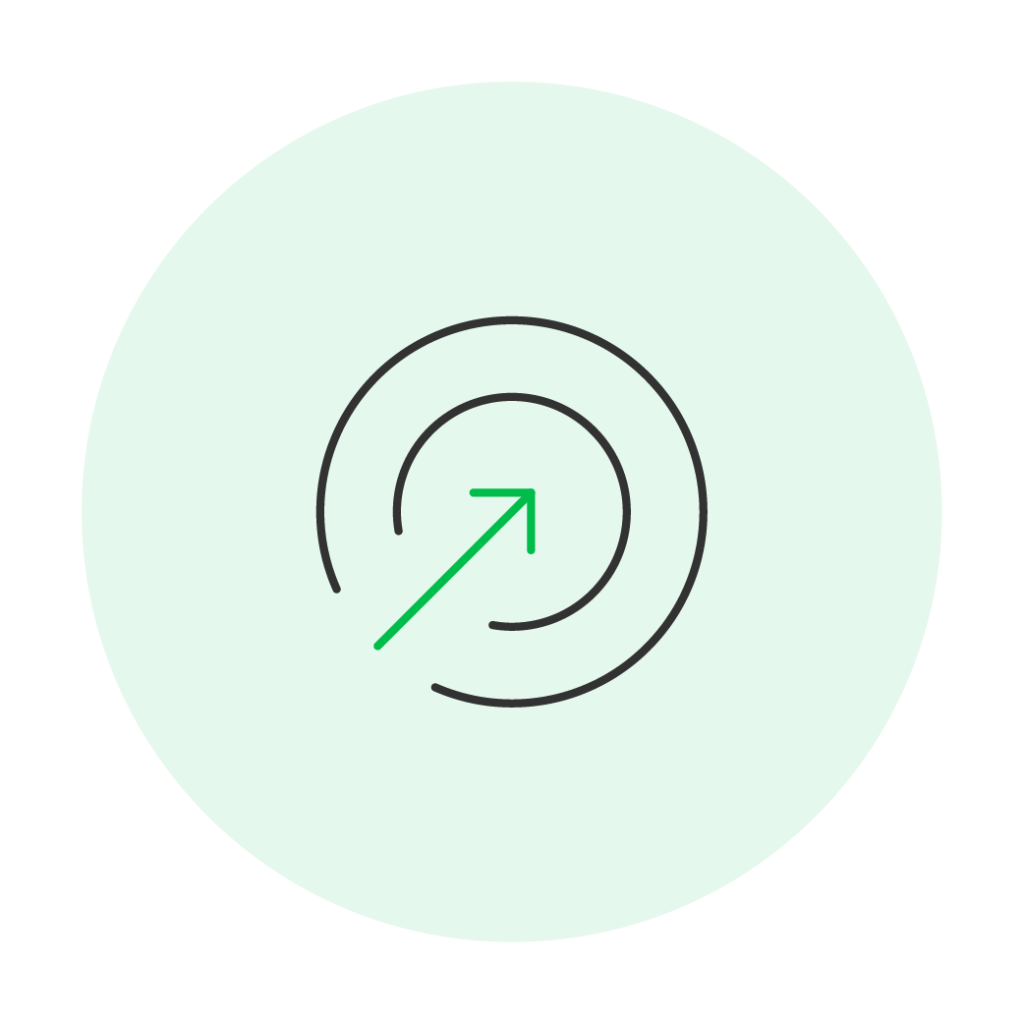
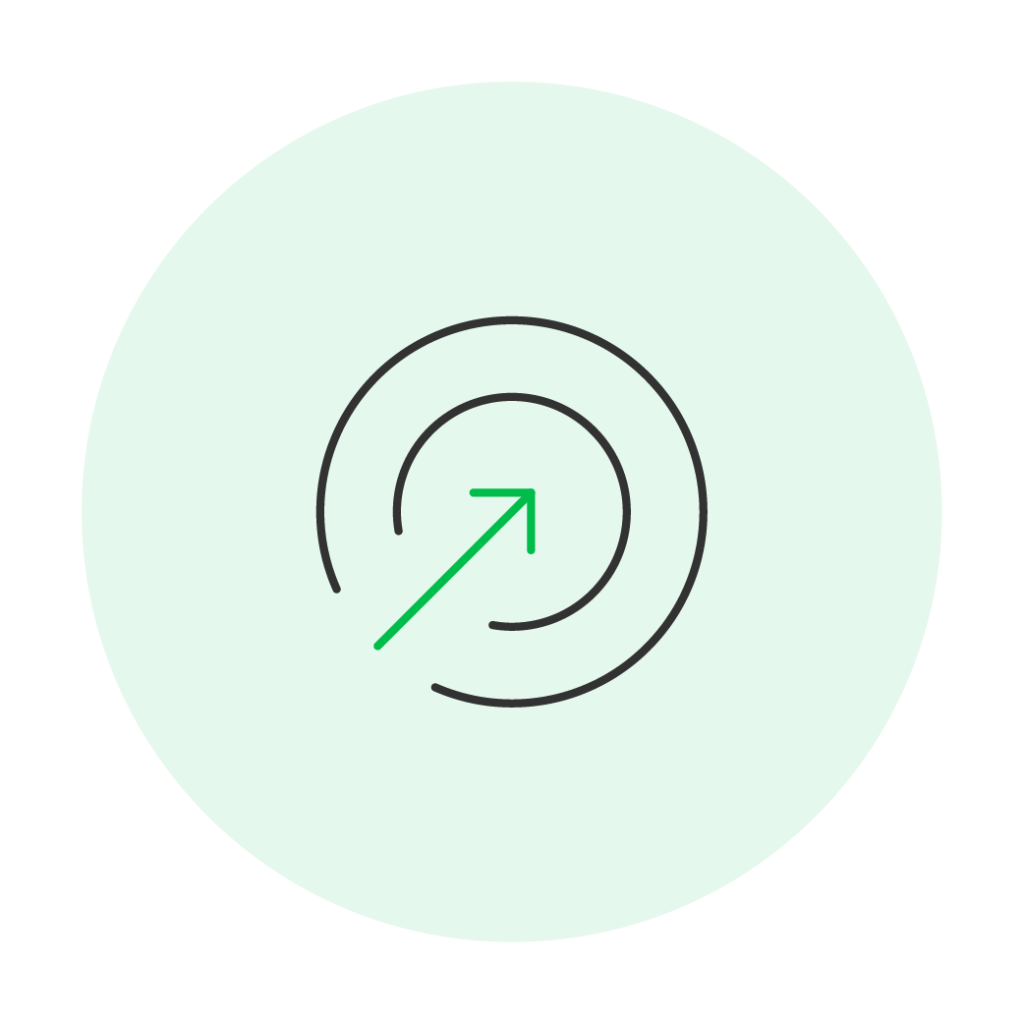
Aim for Production
The ultimate objective of a data science Proof of Concept is to demonstrate the feasibility and value of the proposed solution and pave the way for production deployment. As the PoC nears completion, it is crucial to assess its scalability, robustness, and integration requirements with existing systems.
This evaluation helps in transitioning the successful PoC into a production-ready solution that can be seamlessly integrated into the organization’s operations.
3 Quick Tips
- Evaluate the scalability of the POCs infrastructure, algorithms, and data pipelines to ensure it can handle larger datasets and increased demand.
- Identify any integration challenges with existing systems and prepare a roadmap for seamless deployment and adoption.
- Document the lessons learned and best practices from the PoC to facilitate knowledge transfer and ensure a smooth transition to production.
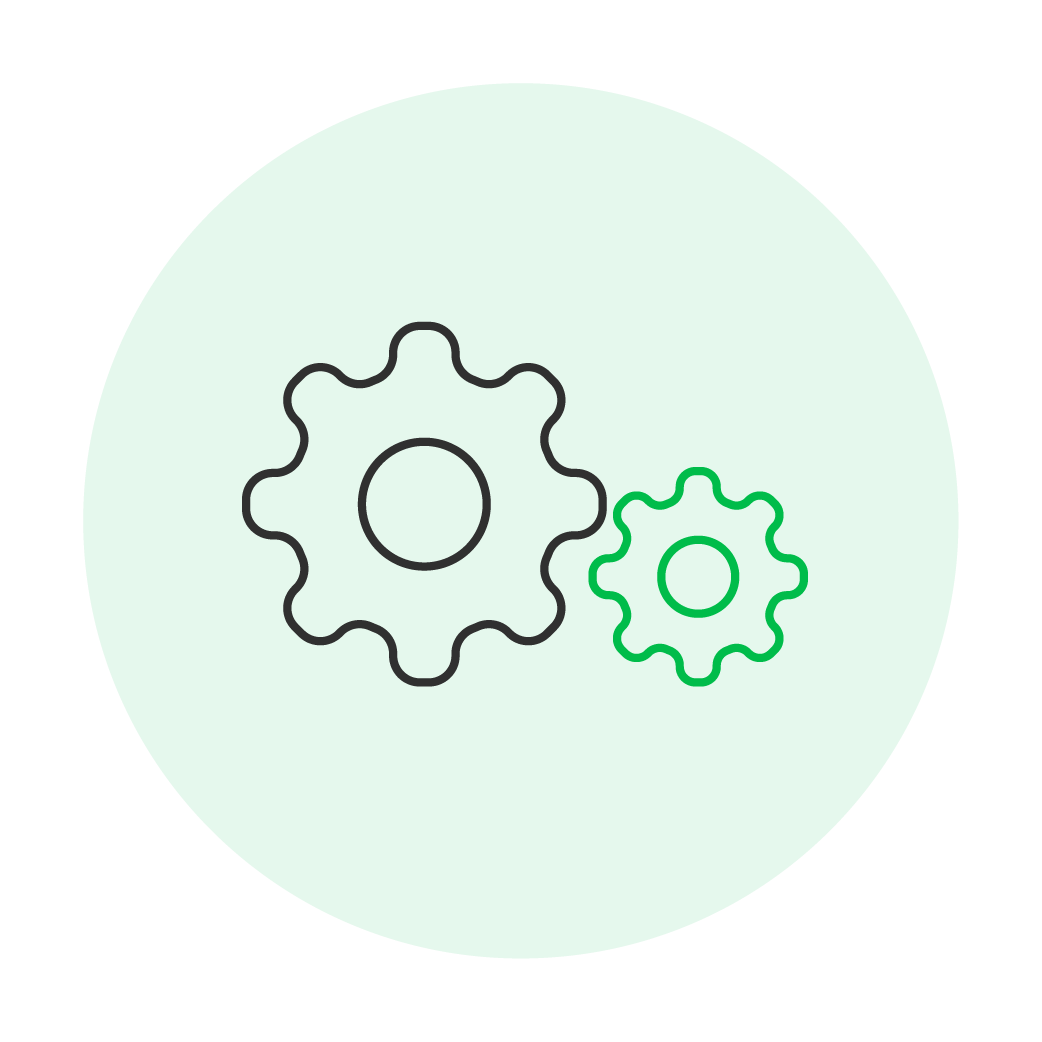
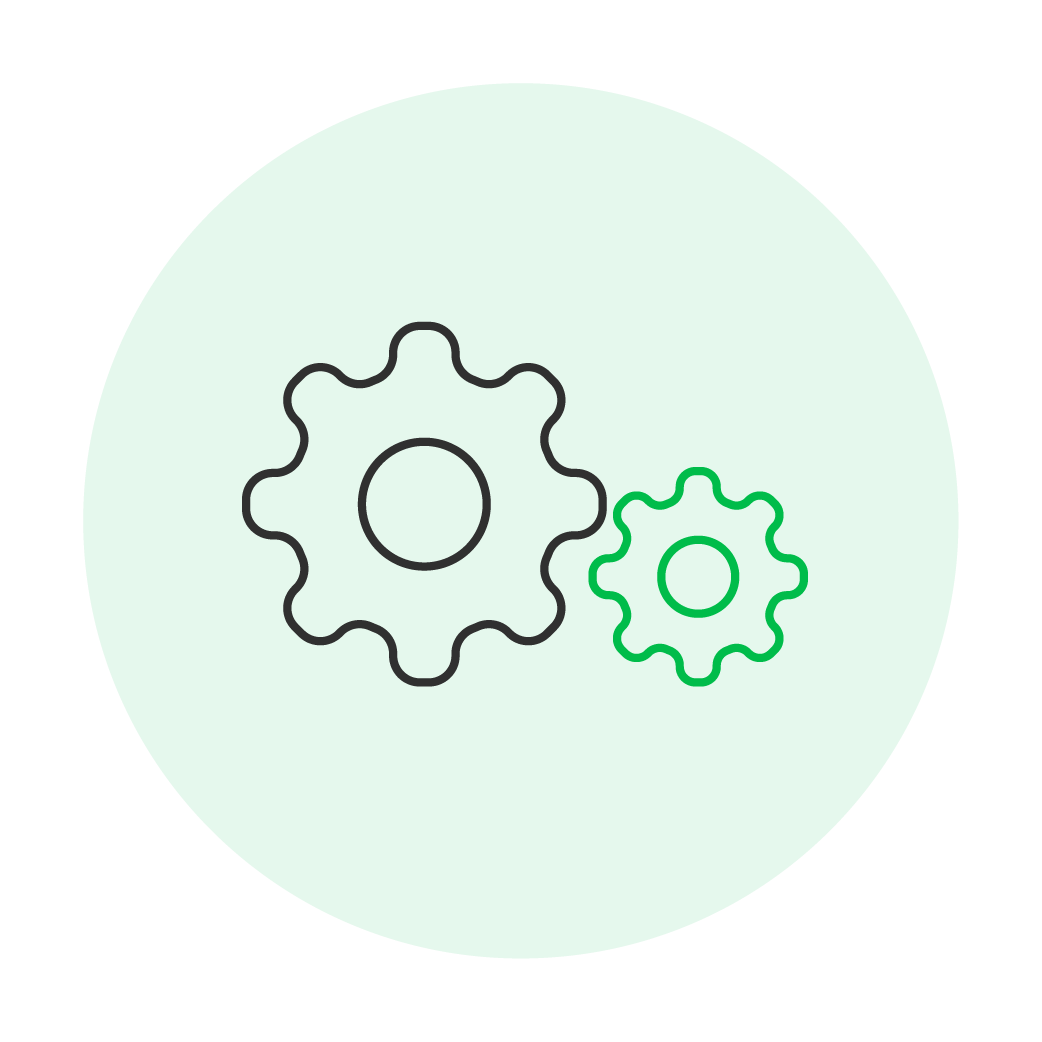
How DataNorth Helps You Realize Successful PoC Projects
Are you looking to minimize the risks of failed Proof of Concept projects? Or are you seeking assistance in starting your own AI PoC but lacking the necessary experience? Look no further than Klippa DataNorth. Our team of expert AI consultants specializes in building and executing Proof of Concepts. With our services, you can mitigate risks and gain early insights into whether your solution aligns with your desired requirements.
Upon completion, we provide a comprehensive product demo along with a detailed report outlining the functionality and offering future recommendations. We prioritize transparency and ensure that you have a thorough understanding of the PoC outcomes.
If you’re interested in discovering how DataNorth can assist you, please feel free to contact us, and our team will be delighted to discuss your requirements in more detail.
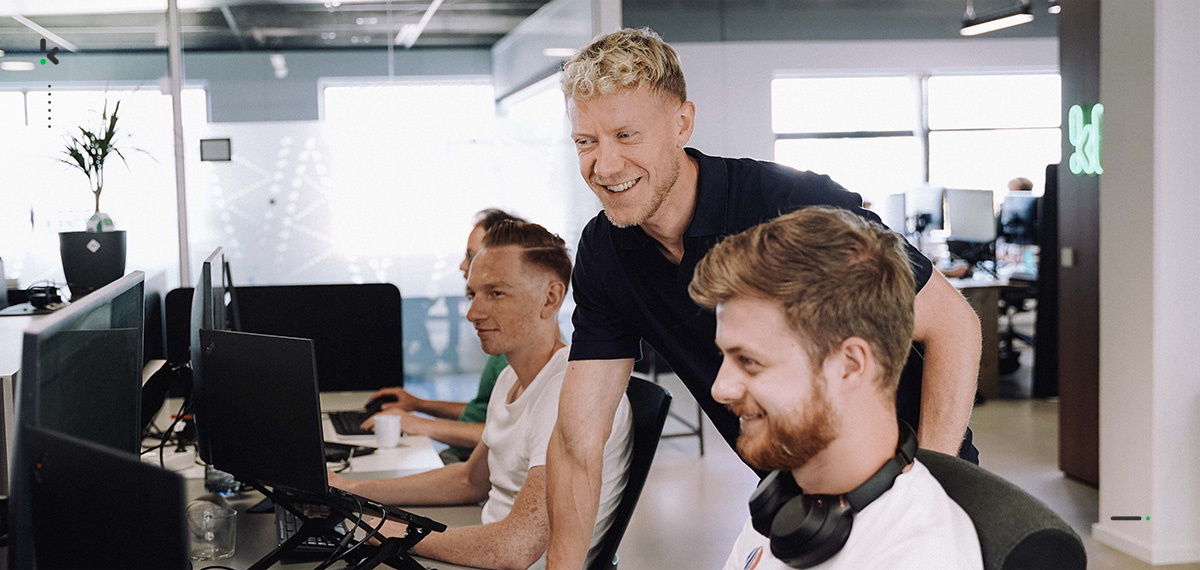
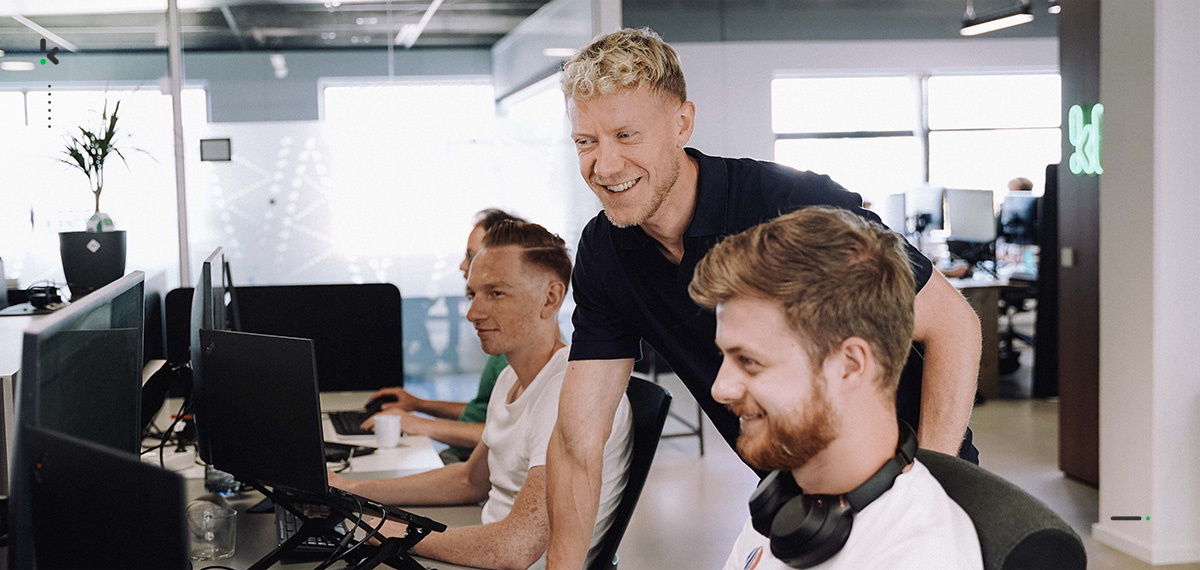