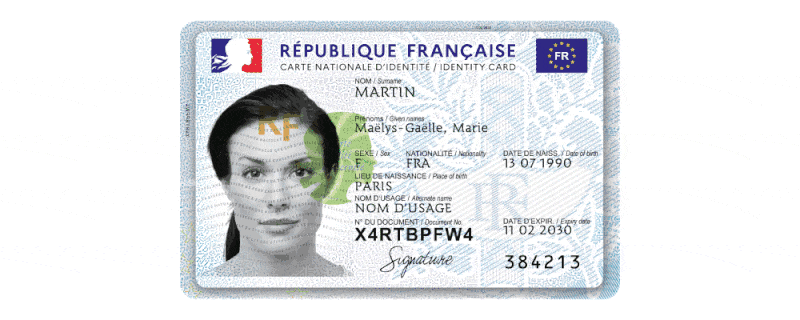
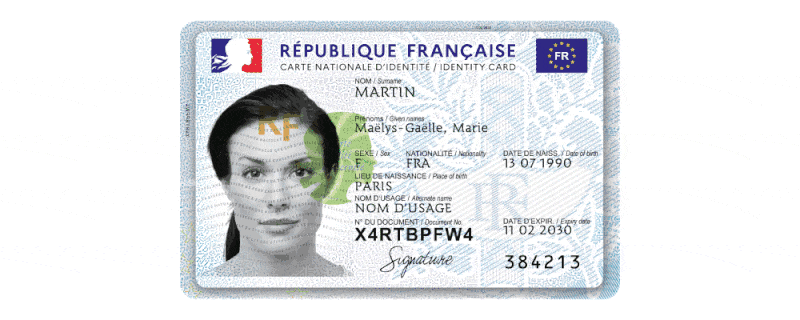
Using an OCR solution is already widely spread. As a matter of fact, in 2021, the global OCR market size was valued at 8.93 billion dollars.
Most companies thus already know the importance of Optical Character Recognition (OCR) in automating document processing. But why is that? The answer is simple. OCR solutions provide an easier, faster, and more efficient way to process documents with little or no human intervention needed. It’s the step companies need to take to stay competitive.
Many of these companies make use of template-based OCR, which works fine if you have to process only one document type in one language. Basically, it works best with one specific structure with no varieties in layout.
However, you might have to process multiple document types, such as invoices, receipts, and passports, in different languages. Template-based OCR can’t handle such documents efficiently as they are unstructured and don’t always follow the same layout.
In such a case, what you would need is an alternative to template-based OCR. An alternative that can help you process unstructured data from a wide variety of documents: Machine Learning OCR.
This article will teach you more about Machine Learning OCR and how this technology can help you move forward. But first, let’s explain in more detail why template-based OCR is only the first step in automating your document processing.
Template OCR, the first step in automating document processing
Template-based OCR is often referred to as traditional OCR. Like any other OCR software, it reads, extracts, and provides output data for further processing. The main particularity of template-based OCR is that it’s trained to work on specific types of documents, formats, and languages.
In addition, it can only work with structured data, such as names, dates, addresses, or stock information in standardized formats. For template-based OCR, they also have to be in the exact same location the software was trained to look for them.
If you are using template-based OCR, we told you probably nothing new so far. You know how it can be used and what it does.
In that case, it’s likely that you’re also aware of the challenges of using template-based OCR, especially when it comes to scalability. With every new document you want to process, you need to create new templates. These templates basically define the rules for the software and where to look for what information.
What if we told you there exists a more advanced alternative? One that is not limited by templates and specific layouts: Machine Learning OCR. In the following section, you’ll learn more about Machine Learning and how it can make your life a lot easier.
What is Machine Learning?
Machine Learning is a branch of AI using mathematical models of data to guide computers to learn without human instructions. To put it simply, Machine Learning enables a machine to replicate intelligent human behavior.
Next to that, Machine Learning continuously learns, gradually improving its accuracy, and makes future predictions by using past and present data.
But what does all of this have to do with OCR? Let’s find out next!
Machine Learning OCR
Machine Learning enables OCR software to understand and recognize the general context of a document. Thanks to the capability of Machine Learning to make predictions, the OCR software doesn’t struggle with the variety of documents it receives. With enough data, it can predict where certain data fields appear and extract the data from documents accordingly.
Of course, a lot of data is needed for the prediction models to be accurate. However, you don’t need to create new templates with strict rules every time you deal with a new supplier or document type.
Next to that, some Machine Learning OCR solutions are able to detect anomalies within text or document structures, which is why they are leveraged to detect document fraud.
Now both technologies have been explained, it’s time to actually see why Machine Learning OCR is the best alternative to template-based OCR.
Template OCR vs Machine Learning OCR
To prove our point of introducing Machine Learning OCR as the best alternative to template-based OCR, we will compare both approaches on the following points:
- Ability to process structured and unstructured data
- Learning capabilities
- Employee involvement
- Accuracy
- Cost and time savings
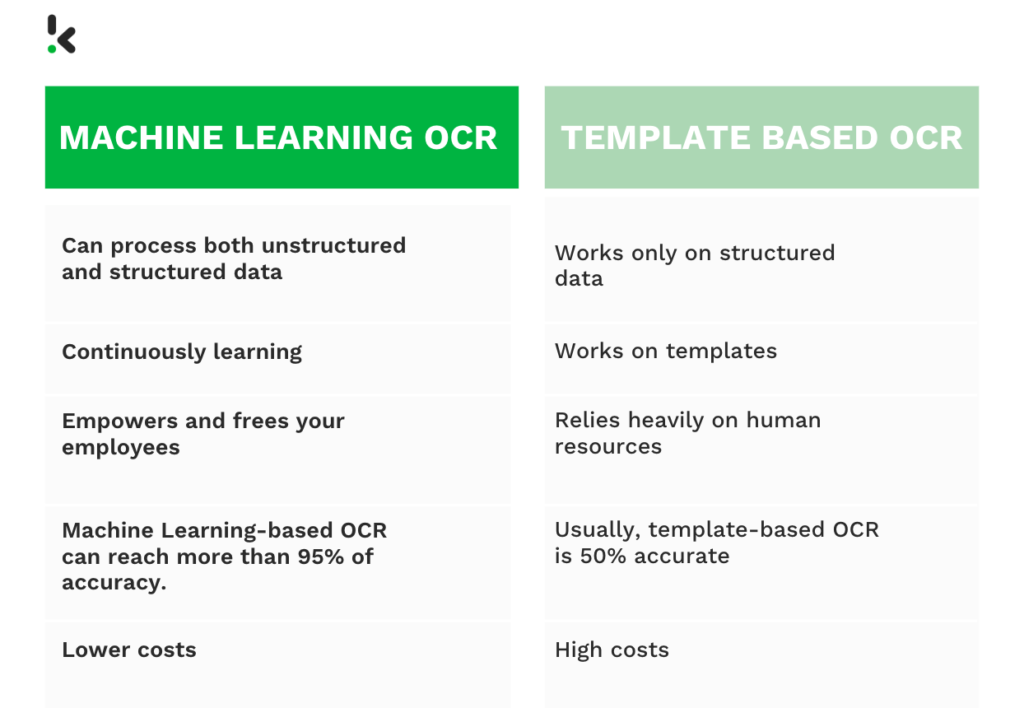
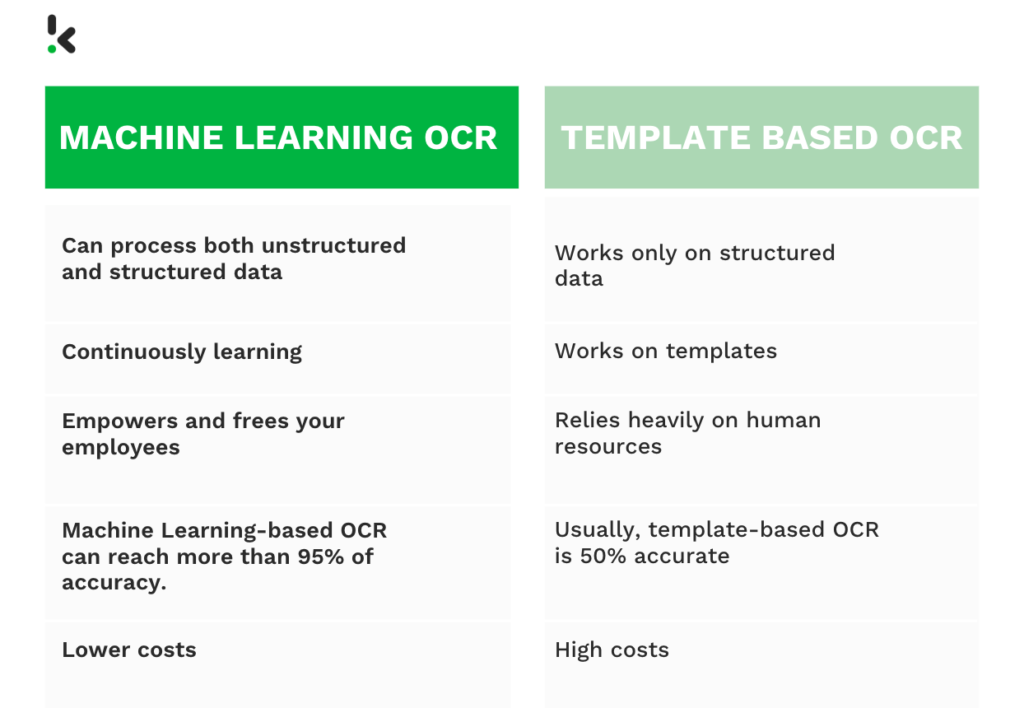
Let’s take each of these points and see why Machine Learning OCR is the best fit when it comes to document processing.
Ability to process structured and unstructured data
Machine Learning OCR can process both structured and unstructured data on a document. Let’s take an invoice as an example. If trained properly, Machine Learning OCR will understand which data are amounts, merchant details, line items, and so on. Not on one specific invoice template, but on every invoice you receive.
Since Machine Learning OCR works with predictions and imitates human intelligence, it can classify documents based on the content and structure. All documents can be processed accurately as long as the engine has been fed enough data.
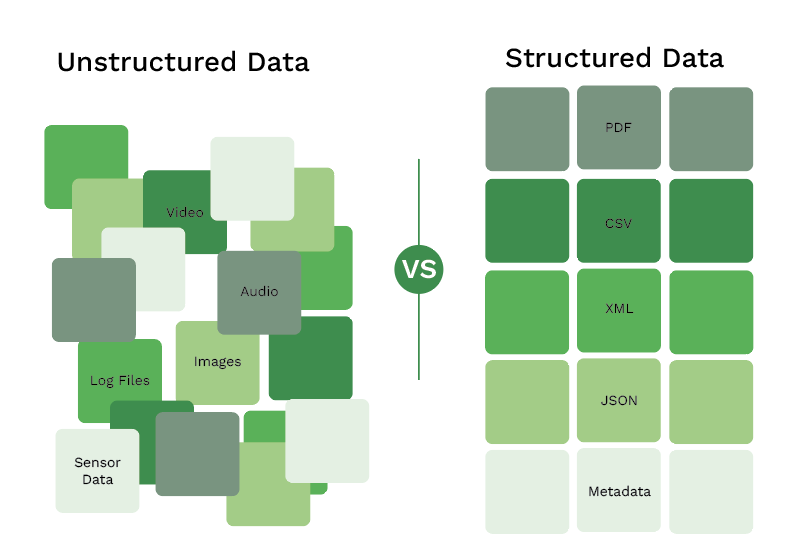
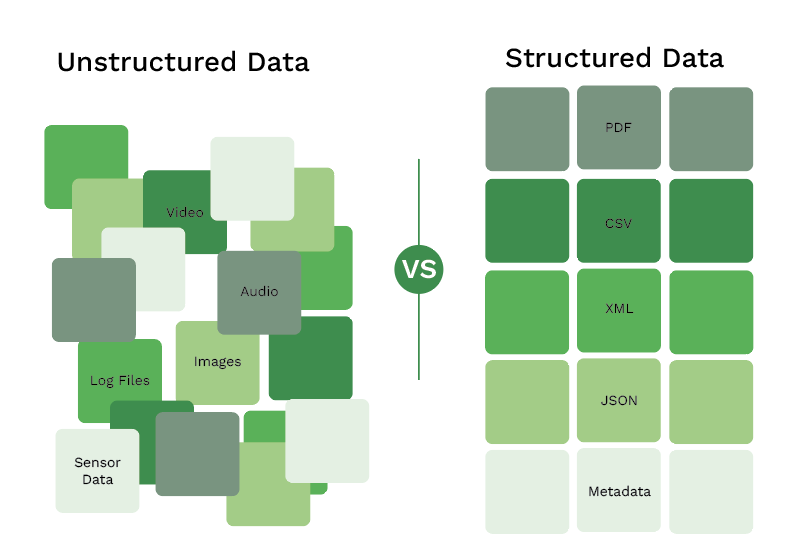
With Machine Learning, you are able to process all kinds of documents whether they contain structured or unstructured data. Template-based OCR, on the other hand, works only on structured data. This is a significant drawback, as it limits your organization’s scalability in document processing.
Learning capabilities
The main goal of Machine Learning is to allow computers to learn autonomously without human involvement. Let’s explain this learning process in more detail.
Machine Learning OCR is based on prediction models, built from algorithms and training data. First, models are created according to all the documents and data sets it has processed. Instead of looking at a specific position on a document, the algorithms predict where data should be according to all the examples it already read and processed.
Based on the experience the engine gained from other documents, Machine Learning OCR keeps on learning. That’s why you need fewer resources to improve it.
With fewer resources needed for improving the OCR solution, your employees can work on more value-adding tasks. Let’s dive into that next.
Employee involvement
Machine Learning OCR can be a big game changer for your company. By automating more processes, your employees are freed from annoying data entry work and they need to be less involved with creating templates for the OCR software. Your team can now focus on more important tasks that contribute to the growth of your company.
So far so good, but what about the accuracy of both approaches? Let’s find out if there’s a difference between the two.
Accuracy
Accuracy is one of the main reasons why companies turn to automation when it comes to data extraction.
Machine Learning combined with OCR technology presents an accuracy rate of more than 95%. To reach this accuracy rate, the Machine Learning model analyzes and interprets raw data. This step enables Machine Learning OCR solutions to recognize patterns and then detect and extract data with high accuracy.
All this information and experience of understanding the document is then used to predict other similarities in the next document.
While conventional OCR, like template-based OCR, has a data extraction accuracy of 60% to 85%, many more advanced solutions embedded with AI and Machine Learning can get up to 99%.
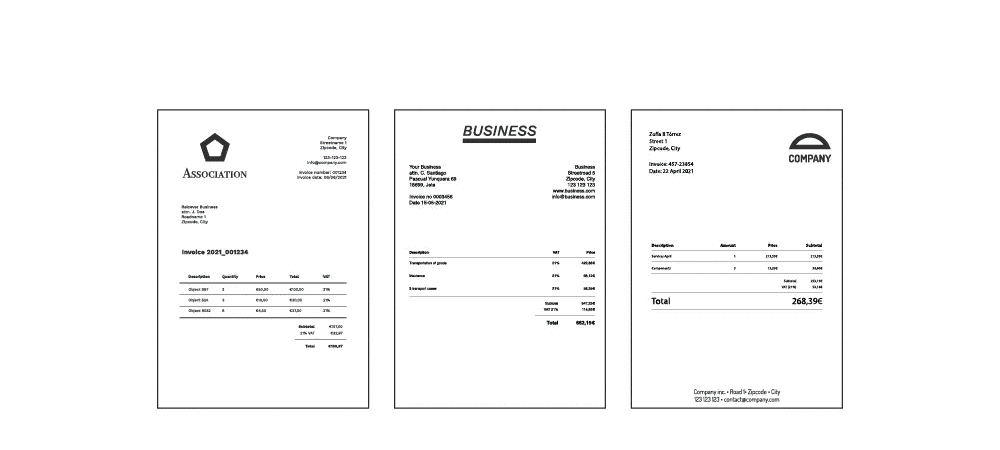
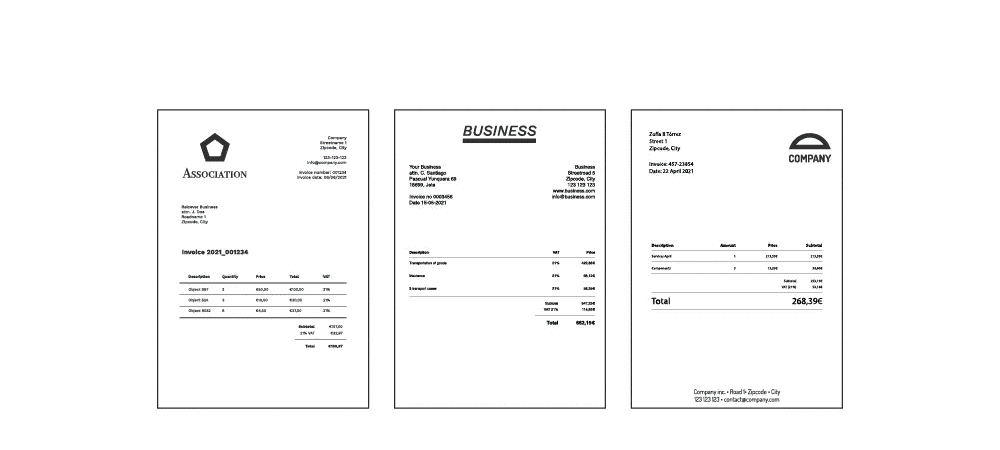
Thanks to Machine Learning, OCR software is almost fully autonomous. It extracts data at a high accuracy rate. This helps you save your team’s time and reduce operational costs. More on that next.
Cost and time savings
In general, Machine Learning OCR is less expensive than template OCR. To prove our point, let’s have a look at the following factors:
- Less human resources required – Higher efficiency leads to lower operational costs.
- Higher accuracy – Less data entry errors save you a large amount of money in the long run.
- No expensive template creation is needed – Saves your organization both time and money.
By now, you learned that traditional OCR is not the most efficient software for data extraction. Using Machine Learning OCR, you can process all your documents faster, with higher accuracy and lower costs.
If you would like to know how Klippa’s Machine Learning OCR can help you achieve that, find more insights below.
Introducing Klippa DocHorizon
At this point, you read the blog and learned about the differences between template-based OCR and Machine Learning OCR. Did we spark your interest in an accurate and efficient Machine Learning OCR solution? Then keep reading, it’s getting even more interesting.
Klippa is an expert in automated document processing. Our company provides intelligent OCR software, such as Klippa DocHorizon, that automates data extraction, classification, verification, and anonymization. All of our software is Machine Learning and AI-based.
Klippa DocHorizon is able to process all kinds of documents: financial documents, ID documents, logistic documents, and so on. Try it out with our examples below or submit a document yourself, and see how our Machine Learning OCR solution performs.
Try it Out Yourself
Within a few seconds, generally between 1 and 5 seconds, the document is processed. Your document is scanned, and all data is delivered in the structured output format of your choice.
Are you ready to automate your document processing? Book a demo with one of our specialists below, they would love to show you the possibilities.